5 Key Components of a Successful Data Knowledge Graph
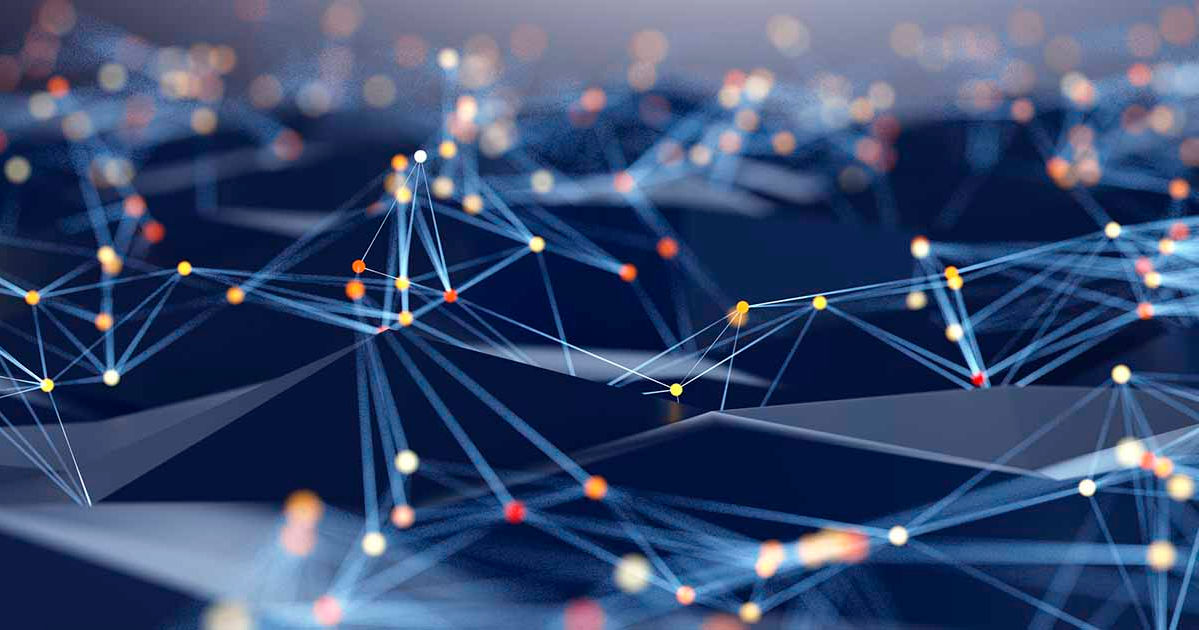
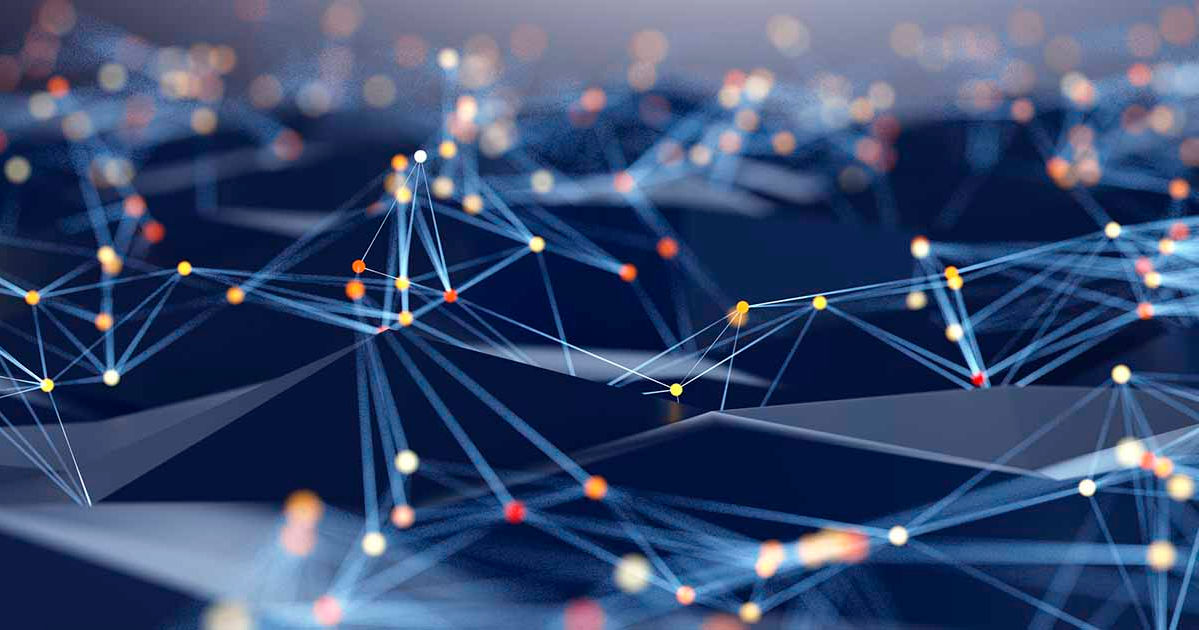
There is a tremendous buzz right now about the need for organizations to develop and maintain Knowledge Graphs. More specifically, there is a growing interest in data knowledge graphs which are knowledge graphs that help to describe an organization's data assets.
A well-maintained data knowledge graph can help answer questions such as:
- Who is using this data?
- Where is this data sourced from?
- How is this data used?
- What depends on this data source?
- Where does this dashboard get its data from?
What is a Data Knowledge Graph?
A knowledge graph is a means to store knowledge in a useful and contextual manner. It helps users quickly find answers to questions that would otherwise require them to search multiple systems, documents or spreadsheets to find an answer.
Knowledge graphs store information as nodes and edges. The node being the subject and the edge being a link to another subject that has something in common. An example would be the "Mona Lisa" is connected to the Louvre and to Leonardo da Vinci.
A data knowledge graph is a repository that stores information about how data assets are used, connected, accessed, and generated . It creates transparency by connecting things such as data sources, reports, dashboards, queries, and users into a standard format. With these connections in place, it becomes easier to understand how these assets depend on each other. They also become easier to discover and reuse.
Why Data Knowledge Graphs Matter
A data knowledge graph provides many benefits to an organization including increased speed, flexibility, and analytical capabilities. Data knowledge graphs allow data and analytics teams to produce insights faster, lending to quicker decision-making and therefore help to make the organization more agile. Data knowledge graphs also help identify new opportunities for revenue generation, margin improvements, and risk reduction.
Additional benefits of having a data knowledge graph:
- Greater reuse: Instead of rebuilding queries or analytical assets, searchable data knowledge graphs allow users to see if someone else has already solved their problem.
- Data lineage: Data Lineage is a pressing concerns when dealing with today's complex data environments. Users need to know where data comes from and when it was last updated. The data knowledge graph can provide a clearer picture of how data has been procured.
- Enhanced security: Armed with a data knowledge graph, the organization can improve its security posture and increase controls by understanding how data assets are accessed and by whom.
- Decreased clutter: Data knowledge graphs make the removal of unused data assets easier since dependencies become more transparent. This helps reduce clutter and also helps to make the data environment easier to secure.
- Impact analysis: Knowing how a data asset connects with other parts of the infrastructure makes implementing change easier. A user will clearly see the upstream and downstream components that could be impacted. This capability is also useful when supporting business continuity and disaster recovery efforts.
- Enabling artificial intelligence: Software vendors and data scientists today are exploring how using metadata can allow for a better understanding of data usage or consumption. Data knowledge graphs empower the creation of predictive models to help developers and analysts find and use the correct data for their given use case. The knowledge graph becomes mandatory for organizations that want to pursue this effort as a clean and consistent data source is critical for the algorithms to be effective.
- Expanded self-service analytics: Placing a Business Intelligence (BI) tool like Power BI or Tableau on top of a complex data environment is only "self-service" for those with the proper credentials and skillsets to leverage these tools. In contrast, data knowledge graphs expand self-service access for everyone else, removing bottlenecks and limitations to quality data access. Without a means to codify knowledge into a consistent semantic layer (aka the knowledge graph), organizations will never truly achieve "self-service" for anyone other than highly skilled specialists.
Now that we know the benefits of having a data knowledge graph, let's discuss the key ingredients to building one.
New to Knowledge Graphs? Catch up on what the other experts are saying:
Knowledge Graphs Become Central Component of the Data Fabric (Gartner)
You Need To Be Thinking in Knowledge Graphs (Forbes)
5 Key Components of A Successful Knowledge Graph
- HAVE A WELL-DEFINED STRATEGY: Building a data knowledge graph requires a well-defined strategy, as technology alone will not solve the challenges caused by a highly disconnected and heterogeneous data environment. The most successful knowledge graph strategies incorporate both technology and process change components. This is because data about data (aka metadata) changes often. A means to capture this change in a clear and consistent fashion is critical. The ability to audit the validity of this information is also important.
- SIMPLIFY ACCESS TO INFORMATION: A data knowledge graph needs knowledge about data! Unfortunately this knowledge is often found in spreadsheets, documents, portals and other squirrely places. A simple method to collect this knowledge into the graph is needed to ensure accuracy and consistency. Workflow capabilities, like those that come with Process Tempo, make it easy to capture this knowledge by providing a simple to use, non-technical UI for those to share what they know.
- DEVELOP A COMMON SEMANTIC DATA LAYER: Defining an upfront set of terms to describe data held by the knowledge graph is critical. Consistent use of terms or phrases prevents chaos. An example might be the definition of a "Customer." A customer can mean different things to different teams and be labeled differently across different datasets. For the data knowledge graph to be successful it must be consistent and simple. Again, platforms like Process Tempo make it easy to define a common set of semantics.
- LEVERAGE GRAPH TECHNOLOGY: Graph databases (like neo4j.com ) are data repositories purpose-built for storing data in graph form. These platforms make it easy to capture and analyze the relationships that a knowledge graph relies on. Developing a knowledge graph using any other type of repository will eventually cause performance, speed, scale, and organization problems. Using the right technology stack will greatly improve future success.
- UTILIZE ADVANCED INTEGRATION: A data knowledge graph will increase in value when it can automatically collect metadata from the organization's existing data platforms. Advanced integration techniques will be required to blend this data into a common standard as these external data platforms may use different identifiers and naming conventions.
A successful data knowledge graph requires these key components. Any other approach will lead to delay, frustration and added cost. To maximize your efforts make sure you start off on the right footing by developing a sound strategy, that access to information is kept simple, that your effort includes a common semantic layer, it leverages a graph database and offers advanced integration capabilities.
Ready to get started?
To learn more about how data knowledge graph solutions can help you organization, or to learn how to develop your own, shoot us an email at info@processtempo.com or talk to a representative . We would be happy to help!
Discover the Power of Data
Unlock insights and drive business growth with our platform
Related News
Discover the latest trends and insights in data analytics.